経営工学系ワーキングペーパー 2017-5 掲載
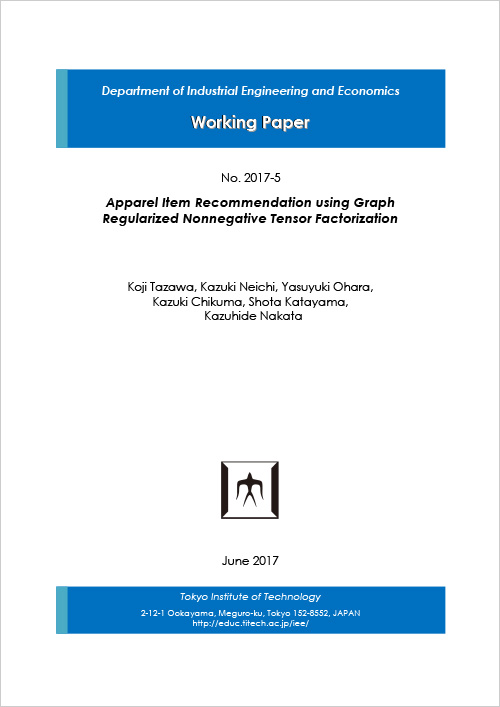
経営工学系ワーキングペーパー 2017-5
経営工学系では、系の最新の研究成果の報告を掲載する「ワーキングペーパー」を発行しております。
最新号は「2017-5:
Koji Tazawa, Kazuki Neichi, Yasuyuki Ohara, Kazuki Chikuma, Shota Katayama, and Kazuhide Nakata, Apparel Item Recommendation using Graph Regularized Nonnegative Tensor Factorization」です。
- 論文タイトル
- Apparel Item Recommendation using Graph Regularized Nonnegative Tensor Factorization
- 著者
- Koji Tazawa, Kazuki Neichi, Yasuyuki Ohara, Kazuki Chikuma, Shota Katayama, Kazuhide Nakata
- 論文要旨
- The number of items in e-commerce sites is continuously increasing, and attributes of those items are becoming more complicated. So it is crucial to find a way to deal with complicated data. A recommendation system using Nonnegative Tensor Factorization, NTF, is one way to tackle the problem. In NTF, we can deal with the data with 3 or more attributes like users, categories, and so on, as tensor's axes. In this paper, we proposed a new enhancement of NTF, named Graph Regularized NTF (G-NTF). The idea is based on one of the enhancements of Nonnegative Matrix Factorization, GWNMF [2]. Its factorization is regularized by graph networks which represent similarities between elements in each attribute respectively. We proved that the simple improvement of GWNMF's updating formula will converge in our method. G-NTF was applied to the real dataset of an apparel e-commerce site. This revealed that our method is successful and can recommend more suitable items to users than any other methods.
今後も新しい号が発行され次第掲載していきます。